A few months ago, François LUCQUET and Anaïs ETIENNE told us of the growing interest in quantifying cyber risks[1], but also warned us against going to the path of quantification without prior reflection. Their analysis, which is still relevant, emphasized in particular the level of maturity required to engage in a method of quantitative estimation. This latter point of maturity level drastically reduces the scope of organizations which are likely try it out. However, some methods of quantification are the source of solutions that give hope in the ability of quantifying its risks in financial terms, and by the same logic of being capable to estimate a return on investment.
It is therefore useful at this point to take a look at the existing methods and the theories that could lead us to concrete results. In the big bang of cyber risk quantification, what are the theoretical foundation for the development of a method? Which ones have succeeded, which ones seem mature? Can we expect in the short or medium term, alternatives to the current quantitative assessment methods?
Roadmap: Risk analysis and quantification: what can we expect of it?
To locate the quantification in the field of risk management, let’s start by clarifying what we are looking for. Within the risk management process, the primary objective is to define an efficient numerical value, illustrating a level of risk (usually a financial cost).
It is therefore, according to the ISO27k standard, only a new risk assessment. Indeed, preceding phases of risk contextualization and identification have no reason to be affected by quantification. The phases of risk treatment, acceptance, supervision or communication, while they will benefit from the results of the quantitative analysis, are unchanged in their workflow. Simply put, it is only question of changing the way each risk is estimated and computed.
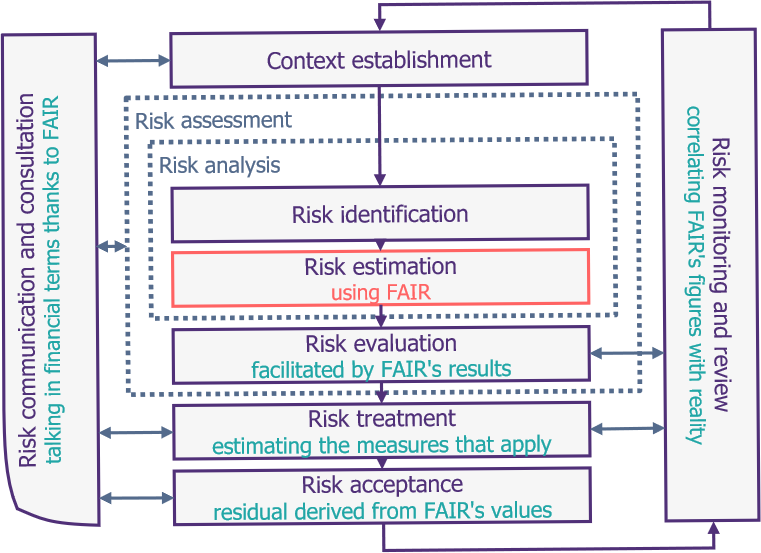
This point, rather trivial but crucial, allows us to ensure that, even if they are fundamentally different from the qualitative methods in their results, the quantitative ones will in any case support pre-existing methods. So, we can be reassured that, although it is necessary to use them to have a mature risk management process, it will also be the basis for the quantification (that will thus exploit the pre-existing risk identification phase).
Now that we have framed the contribution of quantification in an organization’s overall risk analysis, let us specify what we would expect (regardless of the possibility of achieving these assertions):
- On the one hand, it is imperative for this method to be more precise in its result, compared to the qualitative method that it has to replace. This means above all that, from the first occurrence and without having previous results records, it must give a precise numerical estimation (which may as far as possible contain several values: maximum risk or probable risk in particular).
- We may also want it to be faster to achieve (or at least to be carried out in an acceptable time), in order to be able to completely replace the qualitative estimate in the long-term. We are here talking about the time it would take to implement the analysis, without worrying a lot about the time it would take for computations (which can now be efficiently delegated, especially via the cloud). In the end, correlating this with the previous point, it is only question of having a better efficiency than the qualitative evaluation.
- Furthermore, we wish the quantitative assessment to be based on concrete data, in order to gain credibility in the results that will be produced. Indeed, since the workflow of a quantitative method is based on mathematical theories, only an incorrect implementation could introduce subjectivity into the values obtained. This last point would justify that in a time equivalent to qualitative analysis, we have finer results.
- Finally, and this stems from the previous point, we need to have a precise taxonomy, for the collected data to be clearly defined (regardless of the kind of risk). Indeed, if the quantitative estimate is based on proven mathematical theories, the quality of the data produced will then depend only on the quality of the data used as input, and in particular on the relevance and the consistency of the data, depending largely on its definition..
At the core of the galaxy: moving from theory to practice
Having specified what are the characteristics of quantification, let us now see what mathematical theories would take into account the hazard associated with a risk.
Consider, for example, the fuzzy sets theory. This mathematical theory is based on the principle that an element, instead of classically belonging or not to a mathematical ensemble, may only partially belong to it, according to a stated degree. This could be useful to highlight the occurrence or the impact of a risk with the degree of belonging of that risk to ensembles. This theory, while interesting, has not led to concrete applications.
Another approach, which could be called correlative, would be based on the use of self-learning neural networks, to determine from CTI data what the level of risk of a company would be, according to its characteristics. This theory has benefited from the current popularity for artificial intelligence. This led to academics’ studies comparing different modes of machine learning (notably BP[2] or RBF)[3], in order to be used in cyber risk analysis. However, to date, it does not appear mature enough to lead to a realistic method.
Finally, the only mathematical solution that has paid off has been the statistical analysis (and game theory, which offers the means to combine statistical distributions, see the “Risk Quantification and Data: Advice and Tools”[4] article about this subject). The principle of statistical analysis is to rely on statistical observations to estimate the level of a risk. The hazard of risk is then, in large part, taken into account by the distribution of the statistics.
Based on these statistics, two approaches are practicable:
- The first is illustrated by a method proposed by the IMF[5]. It proposes to assess a cyber risk by a detailed statistical analysis. However, it is highly computational and inaccessible for regular use or as a part of a quantified risk estimate. However, it retains an undoubted interest in an analysis of a level of cyber risk on several entities that would have similar data, which may be useful for an insurer or in the banking community. However, it remains confined to this use. Reduced to the already limited scope of entities with acceptable cyber maturity, this method does not seem to be able to offer in the short or medium term an exploitable solution for the IS level of an organization.
- The second is to break down any cyber risk based on common characteristics. This is in particular the approach of the FAIR methodology: it proposes in its taxonomy (see ‘how to apply the FAIR method’1) a dissociation of risk according to its occurrence and the estimated impact, from a financial point of view. FAIR then proposes a declination of these two parameters which, because of their universal nature, may therefore be applied to any cyber risk. This type of method has the advantage of proposing an identical process for the analysis of any cyber risk, facilitating its use in an organizational context (that can then compare cyber risks of distinct natures).
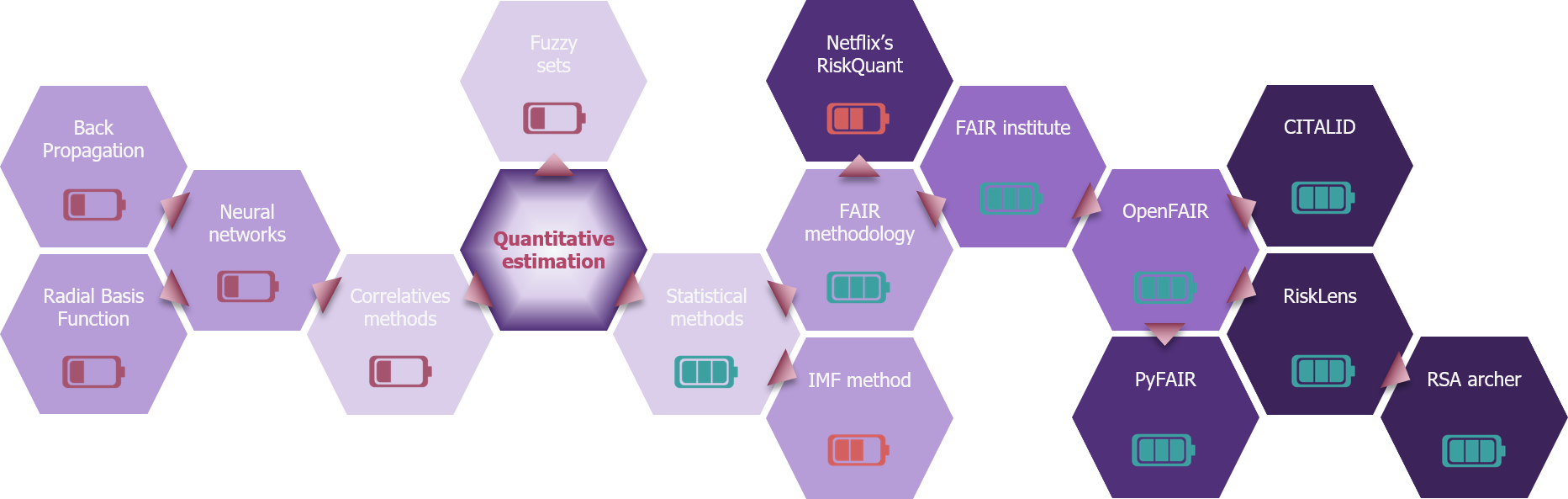
The galaxy of quantification
The FAIR method: a supermassive black hole
Currently, only the FAIR method has risen to applicated quantification solutions for a company. Its monopoly in the field is such that it has become an inescapable reference for a solution or methodology to remain credible. Like a black hole, it attracts to it all the current solutions of quantification. We can, for example, illustrate this with the Risquant Library, developed by Netflix’s R&D department[6]. This one clearly announces that it relies on the FAIR methodology. Nevertheless, he takes great freedom in the interpretation of taxonomy and analysis, but the fact of quoting it allows him to be more easily accepted and recognized.
This hegemony of FAIR can be explained quite easily:
- To begin with, it’s a pragmatic method by design. Its inventor, Jack Jones, set it up when he was an RSSI of a large American group, and was asked to justify cyber ROI. It was therefore initiated for operational purposes, then refined and gained credibility by relying on mathematical tools and theories. This concept of development (i.e. the fact that the method was born out of a need, and then mathematically justified) makes of FAIR a method particularly appreciated by the first concerned, that are the CISO and the other cyber-risk managers.
- Then, it was particularly visionary, as she preceded all other methods. Appeared in 2001, the first book about the method was published in 2006, detailing its operation and taxonomy. As time went on, a community was made up around Jack Jones and his method: the FAIR Institute. This community continued the maturation and thz diffusion of the method. More precisely, it helped developing the efficiency of the method by placing facilitators to make it ever usable.
- The FAIR method also has a particularly solid basis: in addition to the publication mentioned above and which was the subject of an enriched reissue in 2016, it is based on two standardization documents, published by the OpenGroup (the consortium behind the architecture standard of SI TOGAF). The OpenGroup also offers certification to the method, based on its two standards, and which add to the interest laying on the method.
- Finally, FAIR is strongly supported (particularly across the Atlantic): the community that drives it is particularly active, and contributes as much to its evolution as to its promotion: the links between the OpenFAIR and the FAIR Institute, both mentioned above, are substantially close. The strength of his ties is ensured by the fact that Jack Jones, father of the method, plays a central role in both organizations.
Thus, in the world of cyber-risk quantification, the only operational solutions to date all rely on the FAIR methodology, with a more or less large but still displayed parentage.
If the maturity of this method seems now acquired, its monopoly in the field of quantification allows with little doubt to envisage, at least for next years, that it will remain the only method of quantification. In order for another method to be equal, and in addition to the fact that it will have to establish its conceptual credibility, it will above all have to make a place for itself alongside the hegemony of FAIR, while proving that it is more efficient.
[1] https://www.riskinsight-wavestone.com/2020/06/la-quantification-du-risque-cybersecurite/
[2] Back-propagation
[3] Radial basis functions
[4] See the 2nd article on Risk Insight
[5] https://www.imf.org/en/Publications/WP/Issues/2018/06/22/Cyber-Risk-for-the-Financial-Sector-A-Framework-for-Quantitative-Assessment-45924
[6] https://netflixtechblog.com/open-sourcing-riskquant-a-library-for-quantifying-risk-6720cc1e4968