Machine Learning is an emerging topic in recent years, particularly in the context of cyber security monitoring. However, as mentioned in the article “Boost your Cybersecurity thanks to Machine Learning” (Part 1 & Part 2), the development of such solutions requires strong human and financial investments.
Indeed, not all companies have the necessary means (or the will) to develop this type of technology internally, and thus turn themselves to market solutions facing a major problem: how to succeed in quickly choosing and integrating an effective solution in my context?
Why use Machine Learning in Cybersecurity?
The static nature of current detection solutions (antiviruses using signature bases, alert thresholds in a SIEM…) no longer allows to face more and more numerous and varied attacks. In addition, security teams are overloaded by the volume of data to be analyzed.
As explained in the article « Which tools do you need for your SOC? » (Part 2 & Part 3), Machine Learning provides an answer to these problems encountered by the SOC by using behavioral analysis methods to detect advanced attacks and prioritize the alerts to be analyzed.
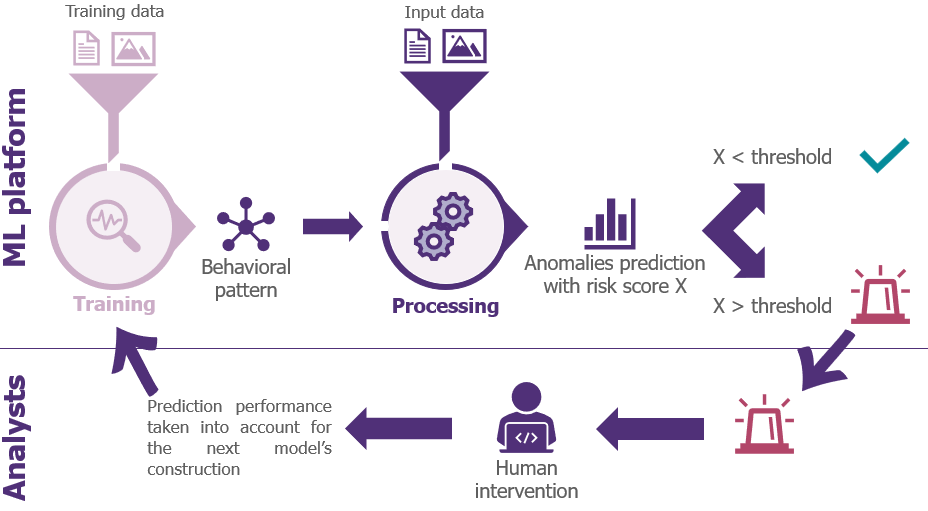
Principle of anomalies detection in a SOC
While these types of solutions provide real added value, they do not completely eliminate the need for current detection methods and are rather used to complement existing tools.
Moreover, their level of complexity (deployment, alerts processing) requires a sufficient level of maturity in terms of detection and reaction (organization, tools, resources, data centralization) before it is relevant to launch a project based on Machine Learning. This will facilitate the scoping phase and speed up deployment.
In advance of phase: defining the specifications
Which use case do I wish to address?
During our various interventions with our clients, we have supported the integration of numerous solutions and we can highlight four main types of use cases on which companies invest:
- Fight against fraud: tools for detecting deviation(s) in user’s behavior(s)
- Email monitoring: prevention tools against phishing or information leakage (DLP)
- Network threat detection: «Next-Gen » probes
- Endpoint threat identification: « Next-Gen » anti-viruses
The choice of a solution (and therefore of a use case) should not be defined unilaterally by the ISS branch, but should be discussed with various stakeholders (ISS, CIO, businesses, etc.). This exchange will enable the target to be specified and the technical and organizational prerequisites to be validated (accessibility of logs, resources to be mobilized, size of teams, etc.) in order to best prepare for its integration and use.
What kind of solution to choose?
Depending on the tools already in place and according to the need, several solutions are possible:
- Choosing to implement a turnkey solution allowing to treat very precise use cases that are not specific to business issues (EDR, behavioral biometrics…). This choice generally suits an immediate need rather than a long-term strategy.
- Activate a Machine Learning module on a tool already in place (SIEM, log sink…) in order to extend its detection perimeter. This choice allows to quickly test use cases and to free oneself from the phases of integration of a new equipment within the IS.
Finally, it is essential to remember that there is no miracle solution and that each type of solution responds to specific needs.
In front of the editor : challenging the essential points
Testing the solution and think about scalability
Once all these prerequisites are defined, it is usual to realize with the editor a Proof of Concept (PoC). However, in the specific case of a Machine Learning solution, the PoC will answer several specific questions:
- Do my currently collected data allow me to have quickly satisfactory results? Machine Learning solutions require the analysis of a very large amount of data potentially enriched by repositories that can be cross-referenced from several sources. It is therefore necessary to make sure in advance with the editor that the data currently collected already allows to obtain first results.
- How long will the learning phase last in my context? Some Machine Learning solutions produce results only after several months or even years because the learning phases can be extremely long due to the specific context of each company. The possibility to use a log history for tests would allow you to free yourself from a significant learning period.
Specific questions will also have to be addressed in order to anticipate the longer term:
- Will it be possible to enrich the analyses with other types of data? Machine Learning solutions allow you to perform analyses on many types of data that may have heterogeneous formats, so it is necessary to be able to ensure that the analyses can be enriched with new types of data collected.
- Will it be possible to implement new detection algorithms? The possibility of being able to customize these solutions by adding new types of algorithms (and potentially independently) is not negligible.
- How can I be sure that my publisher is always at the cutting edge of technology? Given the exponential evolution of techniques on this subject, it is important to ensure that the publisher continues to be at the forefront of technology in order to offer new means of defense against attacks that are becoming increasingly complex.
Preparing to protect the data life cycle
Detection methods based on behavioral analysis require the collection and processing of sensitive/personal data. Thus, especially in the case where the solution is hosted by the editor, issues related to the use of the data will have to be addressed as soon as possible. On the one hand, contractual security requirements will of course need to be reinforced, and on the other hand it may be useful to use upstream solutions that enable more secure processing of the data lifecycle.
For example, startups like SARUS are working on masking personal data, allowing data scientists to perform Machine Learning without accessing source data. Startups like HAZY are working on generating synthetic data that keeps the statistical value of the useful data, but loses its sensitive nature. This type of solution also allows to artificially enlarge the sample provided, and to obtain an almost unlimited amount of data, which can be very useful in the context of a PoC where currently available data is limited.
Once the relevance of the solution is validated, the adventure is just beginning!
Through our various experiences, we have been able to forge a conviction: the market is mature enough to provide interesting results, especially on the four use cases mentioned above. The implementation of such tools will be effective if the solutions are connected to a rich ecosystem and meet a specific need. Indeed, the implementation of one solution can be a success or a failure in two different contexts. The result will depend on the clarity of the need, the scope targeted, the expertise available (Cybersecurity and Data Science), and the availability of the data (quality and quantity).
While choosing a Machine Learning solution is not easy, the best way to get an idea quickly is to realize a PoC that can be quick and involving little engagement: we have seen with some of our customers that solutions were already showing interesting results after only two weeks of PoC.
Keeping in mind that the PoC is only the beginning of the adventure. It will result in the launch of an exciting project lasting several months (analysis of new types of alerts, discovery of new techniques …), bringing a real added value in security (detection of new events …), boosting a new breath within the operational security teams (prioritization of efforts, possibility of optimizing redundant tasks …).