The banking sector has rapidly modernized, and online banking has become a matter of course for both banks and their customers. These players are increasingly reliant on the Internet, with all the advantages that implies, but also the risks.
At the same time, fraud has grown in scale and complexity. According to the Banque de France, payment fraud will represent a loss of 1.2 billion euros by 2022, a considerable sum which is unlikely to diminish as fraudulent transactions continue to increase. Around 70% of these fraudulent transactions come from online banking.
The fight against fraud is therefore one of the most important concerns for online banking, but other sectors are also beginning to address the issue.
Identity fraud, business fraud
The term fraud is part of everyday language and can have a wide variety of definitions. It’s possible to “defraud” a metro ticket, an insurance policy, or a loyalty account with a major retailer.
When it comes to computer fraud, particularly banking fraud, we distinguish between identity fraud and business fraud.
The former involves manipulation of the issuer’s identity data, the context in which he/she accesses the service, or information relating to his/her authentication and authorization. This can be detected by analyzing the user’s authentication behavior, the machine he is using, the IP address from which he is connecting, and so on.
The second involves manipulating data relating to the transaction itself, the banking profile of the sender and receiver, and the context in which the transaction was carried out. Indicators of business fraud could be, for example, a receiving IBAN from an unusual country, a large transaction amount, etc.
The two types of fraud and their detection rely on different signals, but these two protection mechanisms can and must exchange and feed off each other to provide additional context and enable a more holistic analysis of risk.
This need for synchronization has led to a recent organizational rapprochement between business fraud and IAM teams.
What risks are covered by identity fraud detection?
Identity fraud conceals many different uses. Detecting it therefore covers a wide range of risks that are difficult to apprehend today. Here is a non-exhaustive list of techniques used by attackers that could be detected by an anti-fraud tool:
- SIM swapping: SIM swapping involves convincing the victim’s telephone provider to send a new SIM card to the attacker, who can then validate double authentication requests via OTP by pretending to be the victim.
- MFA fatigue: MFA fatigue involves sending a large number of MFA validation notifications, to the point where the victim ends up accepting the request and inadvertently authorizing access to one of their accounts.
- Social engineering: social engineering is used in attacks targeting an individual, where the attacker gathers information about them and their bank account, then exploits it to extract money from them. An increasingly common example is bank advisor fraud, in which an attacker poses as the victim’s advisor and urges him or her to make a bank transfer, often under the pretext of a risk of… fraud.
- Bots: attack automation opens up new possibilities for attackers, who can target a large number of accounts in a single campaign. By emulating devices or launching massive phishing campaigns, it is becoming increasingly easy to recover personal information and passwords.
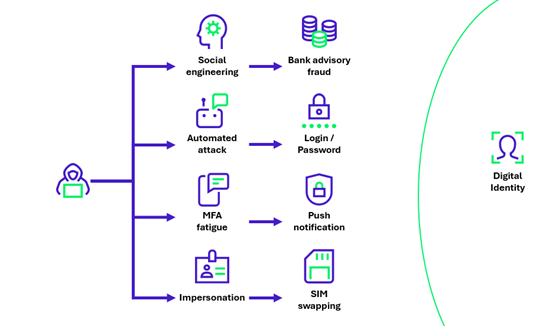
Banks in the lead, but joined by new players
Unsurprisingly, the banking sector has a head start on these issues. Firstly, because the impact of fraud is very real, and the bank is a prime target. Secondly, because users are accustomed to, and even reassured by, significant security processes at the expense of their user experience. Finally, because the massive shift to online banking has raised questions that other sectors didn’t have to ask themselves immediately.
Today, fraud detection for an online bank focuses on three key stages of the user journey:
- Enrolling a new device.
- Validating a payment.
- Performing sensitive actions on the account, such as adding a beneficiary for transfers.
While the banking sector is undoubtedly the most affected and the most protected, other sectors are beginning to address the issue of fraud detection. Retail, e-commerce, and luxury goods, for example, are all in the crosshairs of attackers. This is forcing these sectors to devise new processes and invest in the fight against fraud, in turn driving the evolution of solutions and practices to limit the impact on business.
New technological advances: protocols and algorithms
The pressure of attacks explains much of the interest in fraud detection solutions. These have developed rapidly, embedding more and more functions and demonstrating a growing capacity to combat the complex attacks that are on the rise.
Recent technological advances in fraud detection are manifold, but two main mechanisms have made these solutions more powerful: the ability to exchange information between detection bricks, and the precision of risk estimation algorithms.
The first mechanism is a product of the current trend towards standardization of detection protocols and signals, enabling the various IS bricks to pool the information gathered and the appropriate reactions. The Shared Signals working group (Okta, Cisco, Disney, OpenID Foundation, etc.), for example, has produced a framework used in two protocols: Continuous Access Evaluation Protocol (CAEP) and Risk Incident Sharing and Coordination protocol (RISC).
The second mechanism – the precision of algorithms – is based on the growing number of criteria that can be exploited. A few years ago, a detection engine relied on IP analysis, geolocation and a few identity attributes. Today, the criteria are multiplied, including the user’s own behavior (mouse movements, typing speed), analysis of the devices used (model, OS, browser), account history, common user paths, as well as a panoply of weak signals from other applications or IS bricks. This multiplication of signals entering the algorithms enables a much more refined analysis of each transaction, and an ever more pertinent estimation of risk.
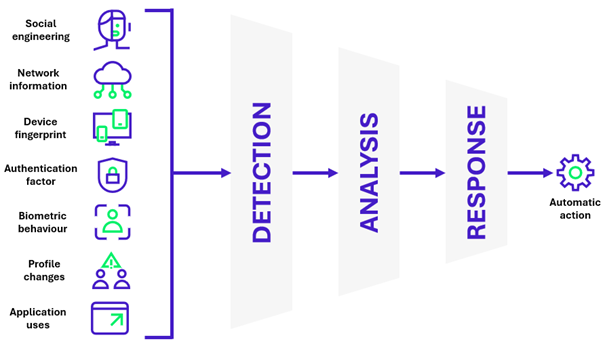
AI and orchestration in the fight against fraud
Increasing the number of criteria helps to improve algorithms, but to get the most out of this information it is essential to take advantage of the capabilities of Machine Learning and artificial intelligence. Each criterion becomes a dimension enabling AI to dynamically learn user behaviours (such as common paths, mouse click locations or typing speed) and what constitutes a normal, non-risky access context, in order to better detect anything that deviates from it.
Despite AI’s ability to produce a decision from a very large number of parameters, it remains a victim of the setbacks of all decision algorithms: false positives. And with the interest of new sectors, which need to balance security and user experience to limit negative impacts on business, the management of false positives is an issue in its own right for software publishers. Today, detection models can be adjusted in several ways: by training them recurrently, to adapt them to new use cases; by playing with the weights of the criteria, according to the customer’s context; and by going back over the decisions taken by the algorithm in order to report false positives.
Beyond these adjustments, fraud detection solutions offer great flexibility in terms of orchestration, i.e. the reaction to be implemented in response to the algorithm’s recommendations. In this way, it is possible to limit the impact on users, by using invisible challenges for low-risk transactions, and by limiting constraining requests such as MFA or deferred manual processing to high-risk transactions. Orchestration also makes it possible to implement the tool progressively: reactions can be limited to raising alerts transmitted to a SIEM tool, for example, to refine the algorithm, then moving on to effective, real-time blocking.
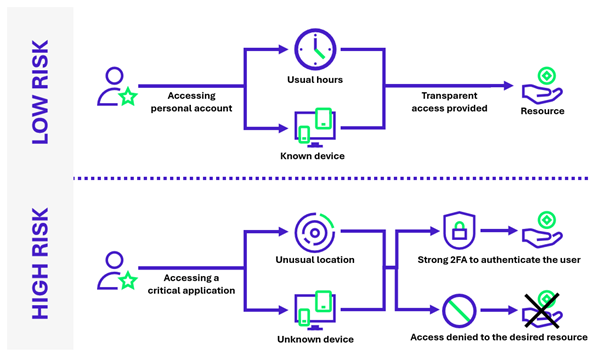
Conclusion
The fight against fraud is a subject that concerns many sectors. While the banking sector is ahead of the game, with e-commerce and luxury goods following suit, any organization can be targeted by fraud. This implies a wide range of use cases and issues to which fraud detection solutions can often, but not always, respond.
The sector of activity, the context, the recurrence and type of attacks, the impact and associated risk, as well as the resources that can be deployed – all these dimensions need to be taken into account to contextualize countermeasure solutions. These solutions may be expensive or unsuitable, despite the innovative mechanisms put in place, and other remediation mechanisms may need to be considered depending on the context.
This is the case with anti-bot solutions, for example, or risk-based authentication mechanisms, or simply the redesign of certain business processes to make them intrinsically more resilient to fraud. These remedies can accompany a fraud detection solution or be sufficient to counter the cases of fraud observed in the context studied.